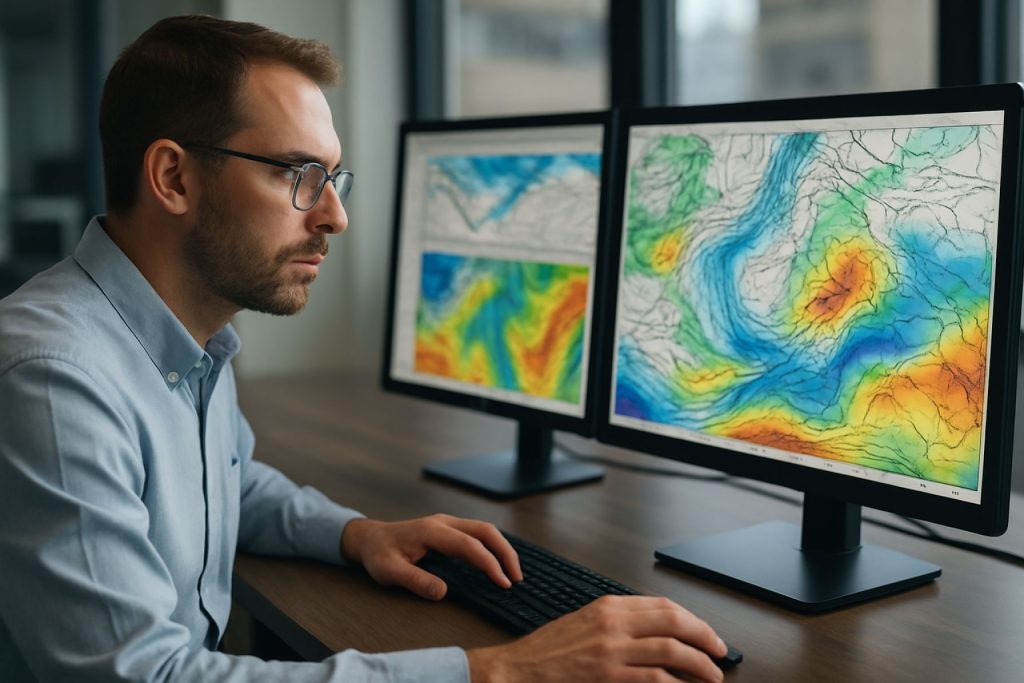
Table of Contents
- Executive Summary: State of NWP Micro-Model Calibration in 2025
- Market Size, Growth Projections & Key Drivers (2025–2030)
- Core Technologies and Calibration Methodologies
- AI, Machine Learning, and Data Assimilation Innovations
- Leading Players, Collaborations, and Industry Initiatives
- Emerging Standards and Regulatory Landscape
- Case Studies: Real-World Implementation and Impact
- Challenges: Data Quality, Model Bias, and Computational Demands
- Competitive Landscape & Strategic Opportunities
- Future Outlook: Disruptive Trends and Market Forecasts Through 2030
- Sources & References
Executive Summary: State of NWP Micro-Model Calibration in 2025
In 2025, Numerical Weather Prediction (NWP) micro-model calibration stands at a pivotal crossroads, shaped by rapid advances in computational power, the proliferation of high-resolution observational data, and the integration of artificial intelligence (AI) methodologies. NWP micro-models—localized, fine-scale representations of atmospheric processes—are increasingly essential for delivering precise, short-term weather forecasts, especially as climate variability intensifies and local-scale events become more impactful. The calibration of these models, which involves tuning model parameters to enhance predictive accuracy against real-world data, has become a focal point for meteorological agencies, technology providers, and academic institutions worldwide.
The past year has witnessed unprecedented collaboration among leading weather agencies and technology firms to address calibration challenges. For example, agencies such as European Centre for Medium-Range Weather Forecasts and National Oceanic and Atmospheric Administration have expanded their investments in cloud-based supercomputing and data assimilation systems, enabling more frequent and detailed model updates. Concurrently, hardware manufacturers like NVIDIA Corporation have introduced new GPU architectures tailored for high-throughput weather model training and calibration, dramatically reducing the time required for iterative optimization.
A key trend shaping the state of NWP micro-model calibration in 2025 is the incorporation of machine learning algorithms into traditional calibration workflows. These AI-driven methods allow for real-time adjustment of model parameters by leveraging both historical and streaming observational data from remote sensing networks, including satellites and ground-based sensors. This integration has led to notable improvements in forecast skill at hyperlocal scales, with urban microclimates and extreme weather phenomena—such as flash floods and urban heat islands—now being modeled with increasing confidence and granularity.
Looking ahead, the next few years are expected to bring further automation and democratization of calibration processes. Open-source platforms and cross-institutional data sharing initiatives are lowering barriers for smaller meteorological services and private sector innovators to participate in model development and calibration. Moreover, strategic partnerships between public weather agencies and private technology companies, exemplified by collaborations involving IBM Corporation and Microsoft Corporation, signal a future where hybrid cloud-AI ecosystems play a central role in operational weather prediction.
In summary, 2025 marks a transformative period for NWP micro-model calibration, characterized by technological convergence, enhanced data flows, and collaborative innovation. These advances are expected to drive even greater forecast accuracy and resilience, supporting critical sectors from emergency management to agriculture in the face of evolving weather risks.
Market Size, Growth Projections & Key Drivers (2025–2030)
The market for Numerical Weather Prediction (NWP) micro-model calibration is undergoing significant transformation as precision forecasting becomes integral to sectors like agriculture, energy, disaster management, and urban planning. Entering 2025, the global demand for highly localized, real-time weather insights is driving investments in micro-model calibration tools, which are essential for improving the accuracy of high-resolution NWP models. This demand is further bolstered by the proliferation of IoT sensor networks, remote sensing technologies, and the adoption of machine learning algorithms for model optimization.
The market size for NWP micro-model calibration solutions is difficult to isolate due to its integration within the broader weather analytics and forecasting industry. However, ongoing expansion in the deployment of advanced weather models by national meteorological agencies and private firms points to robust growth. For instance, leading providers such as IBM (via The Weather Company), Developmental Testbed Center (which supports the U.S. Unified Forecast System), and European Centre for Medium-Range Weather Forecasts are accelerating their investments in micro-scale model calibration and validation infrastructure. The increasing number of high-performance computing (HPC) installations dedicated to weather simulation—such as ECMWF’s ongoing upgrades and expansion plans through 2025 and beyond—reflects this trend.
A key growth driver is the intensifying frequency and impact of extreme weather events, spurring governments and enterprises to refine their predictive capabilities and risk assessments. The energy sector, in particular, is a major catalyst, as renewables integration and grid management require granular, location-specific weather forecasts for wind and solar production. Similarly, urban planners and emergency services are adopting micro-calibrated NWP models to enhance resilience strategies, further expanding the market’s scope.
From a technological perspective, the adoption of artificial intelligence and data assimilation techniques is rapidly improving the efficiency and precision of micro-model calibration. Companies such as IBM are leveraging AI to enhance local model tuning, while collaborations between government agencies and research centers are leading to the development of open-source calibration toolkits and standardized workflows. This collaborative approach is expected to lower entry barriers and accelerate market growth through 2030.
Looking ahead, the market for NWP micro-model calibration is projected to experience steady double-digit growth rates, propelled by digitalization, regulatory emphasis on climate resilience, and advances in computational power. As the ecosystem matures, partnerships between technology firms, meteorological agencies, and sector-specific end users will remain pivotal in shaping the trajectory of this specialized market segment.
Core Technologies and Calibration Methodologies
The calibration of micro-models within Numerical Weather Prediction (NWP) systems is undergoing significant evolution in 2025, driven by advances in sensor networks, increased computational power, and the proliferation of high-resolution local data. Micro-models—small-scale, high-resolution atmospheric models—are increasingly being deployed to generate hyper-local forecasts for urban areas, renewable energy sites, and critical infrastructure, where traditional mesoscale models lack sufficient granularity.
Central to micro-model calibration is the assimilation of dense observational data. Recent initiatives leverage expanded urban sensor arrays, advanced weather radar, and IoT-based meteorological stations to provide real-time, site-specific data inputs. For instance, Vaisala has deployed compact weather stations and road weather sensors in metropolitan areas worldwide, offering continuous datasets essential for localized calibration processes. These datasets enable dynamic tuning of micro-physical and boundary layer parameterizations within the models, addressing challenges such as heat island effects and rapid convective developments.
Machine learning is increasingly integrated into calibration workflows. NWP solution providers such as DTN and Meteomatics are incorporating AI-driven data assimilation and error correction techniques, which automatically adjust model parameters based on both historical performance and real-time ground truth data. This approach accelerates the feedback loop between model output and observed conditions, substantially improving short-term forecast skill at neighborhood scales.
In 2025, calibration methodologies are also adapting to utilize crowd-sourced and unconventional data streams. Smartphone pressure readings, vehicle-based weather sensors, and citizen weather stations have become valuable calibration inputs, particularly in data-sparse regions. Companies like Netatmo and WeatherFlow support integration of distributed sensor networks into NWP micro-model frameworks, broadening spatial and temporal data coverage.
Looking ahead, the next few years will see further refinement of ensemble calibration techniques—where multiple micro-model runs, initialized with slightly varied parameters, are statistically combined and calibrated against dense observation networks. This ensemble approach, endorsed by organizations such as European Centre for Medium-Range Weather Forecasts (ECMWF) for broader models, is now being adapted for micro-models to better quantify uncertainty and improve reliability at local scales.
As urbanization intensifies and demand for precision forecasting grows, micro-model calibration will remain a focus of research and commercial development. Enhanced data assimilation, AI-powered error correction, and collaborative sensor networks promise to further elevate the accuracy and value of hyper-local weather prediction through 2025 and beyond.
AI, Machine Learning, and Data Assimilation Innovations
In 2025, the field of numerical weather prediction (NWP) continues to experience rapid advancement in micro-model calibration, primarily driven by innovations in artificial intelligence (AI), machine learning (ML), and advanced data assimilation techniques. Micro-model calibration refers to the fine-tuning of high-resolution, localized weather prediction models to improve accuracy at small spatial and temporal scales. This is increasingly critical as demand grows for precise forecasts in sectors like agriculture, renewable energy, and urban planning.
Recent efforts by key meteorological organizations and technology companies are focused on leveraging large-scale AI and ML frameworks for optimizing NWP micro-models. For instance, European Centre for Medium-Range Weather Forecasts (ECMWF) is expanding its use of machine learning-based data assimilation, integrating more diverse and higher-frequency observational data into localized forecast models. This enables more accurate calibration through real-time analysis and correction of model biases.
Similarly, Deutscher Wetterdienst (DWD) and Met Office are piloting next-generation AI-driven micro-models that can self-calibrate using adaptive learning algorithms. These systems dynamically adjust model parameters in response to the influx of new radar, satellite, and sensor data, thereby reducing the time and expertise required for manual tuning. This approach is expected to become an industry norm by 2026 as computational resources and cloud-based platforms become more accessible.
Tech collaborations are also shaping the landscape. IBM and Microsoft are working with national weather agencies to operationalize cloud-based ML pipelines, enabling near-real-time model retraining and micro-calibration at scale. These pipelines facilitate the ingestion of heterogeneous data streams—including IoT, crowdsourced, and remote-sensing data—improving both the spatial resolution and reliability of localized forecasts.
Looking ahead, the outlook for NWP micro-model calibration is robust. Initiatives such as the NOAA Earth Prediction Innovation Center (EPIC) are expected to release open-source tools and benchmarks for AI-driven calibration workflows, accelerating global collaboration and model transparency. Additionally, the upcoming deployment of new satellite constellations and sensor networks will further enrich the data ecosystem, allowing calibration algorithms to operate with unprecedented granularity.
In summary, 2025 marks a pivotal year in the transition of NWP micro-model calibration from traditional, expert-driven approaches to highly automated, AI-augmented pipelines. This will enable faster, more accurate, and scalable weather prediction services, with tangible benefits anticipated across climate-sensitive industries worldwide.
Leading Players, Collaborations, and Industry Initiatives
Numerical Weather Prediction (NWP) micro-model calibration continues to attract significant attention among industry leaders and research institutions. As high-resolution, localized forecasting becomes critical for sectors like agriculture, renewable energy, and disaster management, the demand for precise calibration techniques and collaborative innovation is driving progress in 2025 and beyond.
European Centre for Medium-Range Weather Forecasts (ECMWF) remains a central player, with ongoing projects focused on improving micro-scale NWP model calibration through advanced data assimilation strategies and ensemble-based uncertainty quantification. In 2025, ECMWF is deepening collaborations with national meteorological services and technology partners to refine localized calibration workflows, especially for urban and coastal microclimates.
Another major organization, the UK Met Office, has been expanding its partnerships with universities and private sector actors to co-develop adaptive calibration frameworks. These frameworks incorporate machine learning algorithms to dynamically adjust model parameters based on real-time sensor data, increasing forecast reliability for critical infrastructure operators and government agencies.
The National Oceanic and Atmospheric Administration (NOAA) in the United States is spearheading initiatives in 2025 to integrate dense observational networks (including IoT weather sensors) for urban-scale NWP calibration. NOAA’s collaborations with technology suppliers and platform providers are expected to enhance rapid model updating and calibration, leveraging cloud-based computing resources to scale real-time weather prediction at the city block level.
Private sector companies are also advancing the calibration landscape. IBM continues to invest in its weather modeling division, leveraging AI-driven calibration techniques to improve hyper-local forecasting. Their collaboration with utility companies and smart city projects demonstrates the commercial relevance of micro-model calibration for optimizing grid management and public safety responses.
On the technology supplier side, Vaisala is providing high-precision environmental measurement instruments and calibration services to support both public and private NWP model operators. In 2025, Vaisala is expanding partnerships with atmospheric modeling consortia to facilitate the seamless integration of observational data into calibration cycles.
Looking ahead, the next few years are poised to see intensified industry-academic consortia, standardization initiatives, and open data platforms that promote interoperability for NWP micro-model calibration. With the proliferation of edge computing and sensor networks, leaders like ECMWF, NOAA, and technology innovators are expected to accelerate the deployment of accurate, micro-scale weather prediction solutions across multiple geographies and economic sectors.
Emerging Standards and Regulatory Landscape
The calibration of micro-models in numerical weather prediction (NWP) is entering a transformative period in 2025, shaped by both technological advancements and evolving regulatory frameworks. As weather forecasting becomes more granular—down to urban microclimates and hyper-local events—the demand for reliable calibration standards has intensified. This is particularly relevant as NWP models now integrate data from distributed sensor networks, IoT devices, and new-generation weather satellites, requiring harmonized approaches to ensure consistency and accuracy.
In 2025, several international bodies are advancing work on standards for NWP micro-model calibration. The World Meteorological Organization (WMO) has continued to update its guidelines on model evaluation and verification, promoting best practices for integrating localized sensor data, bias correction procedures, and uncertainty quantification. These guidelines are increasingly referenced by national meteorological agencies and serve as a baseline for regulatory compliance. Similarly, the European Centre for Medium-Range Weather Forecasts (ECMWF) is piloting frameworks for benchmarking micro-model outputs against high-resolution observational networks, a step toward standardized calibration metrics across member states.
In the United States, the National Oceanic and Atmospheric Administration (NOAA) is updating requirements for calibration and validation of localized NWP products, reflecting the growing importance of micro-models for urban resilience, agriculture, and disaster risk reduction. NOAA’s partnership with private sector technology providers is fostering interoperable calibration tools that can be adopted industry-wide. Commercial weather service providers are also actively participating in these regulatory dialogues, as alignment with emerging standards is anticipated to become a prerequisite for government contracts and public dissemination of forecasts.
Looking ahead, new standards are poised to address challenges such as model explainability, reproducibility, and the blending of data-driven and physics-based calibration approaches. Regulatory agencies in Asia-Pacific are expected to follow suit, with initiatives from the Japan Meteorological Agency and the Australian Bureau of Meteorology indicating a global convergence toward harmonized practices. The next few years will likely see the publication of formal calibration protocols, certification schemes for micro-model providers, and the establishment of open benchmarking datasets. As micro-models become integral to critical infrastructure planning and climate adaptation, adherence to rigorous and transparent calibration standards will be essential for trust and operational reliability in the sector.
Case Studies: Real-World Implementation and Impact
In recent years, the calibration of micro-models in Numerical Weather Prediction (NWP) has transitioned from academic research to real-world operational impact, driven by the increasing need for hyperlocal forecasts in sectors such as agriculture, energy, and urban planning. As of 2025, several organizations are deploying advanced calibration techniques to enhance the accuracy and reliability of their NWP systems.
One notable example is the ongoing work by European Centre for Medium-Range Weather Forecasts (ECMWF), which has integrated high-resolution, localized calibration frameworks into its ensemble prediction systems. By leveraging vast observational datasets and machine learning approaches, ECMWF can fine-tune micro-model parameters to specific geographic regions, resulting in improved temperature, precipitation, and wind forecasts at neighborhood scales.
Similarly, Met Office in the United Kingdom has implemented data assimilation and micro-model calibration techniques as part of its convective-scale NWP upgrades. Their current systems ingest data from local weather stations, urban sensor networks, and even citizen science initiatives, enabling iterative calibration that adapts to evolving microclimates throughout the country. This has had a demonstrable impact on the accuracy of nowcasting, particularly for high-impact weather events such as flash flooding.
In the private sector, companies like IBM (through its Weather Company division) have adopted micro-model calibration within their proprietary forecasting platforms. By coupling real-time data ingestion with AI-driven calibration routines, IBM delivers personalized and hyperlocal weather forecasts to clients in aviation, logistics, and retail, optimizing operational decisions and risk management in near real-time.
Looking ahead to the next few years, the outlook for NWP micro-model calibration is marked by increasing integration of high-resolution remote sensing data, such as data from commercial satellite operators and dense IoT sensor networks. Organizations like National Oceanic and Atmospheric Administration (NOAA) are actively exploring the fusion of these data streams to continuously recalibrate micro-models, aiming for unprecedented spatial and temporal detail in local forecasts. As computational resources and AI methodologies advance, the expectation is for micro-model calibration to become even more automated, dynamic, and responsive, further narrowing the gap between forecast models and real-world weather phenomena.
Challenges: Data Quality, Model Bias, and Computational Demands
Numerical weather prediction (NWP) micro-model calibration faces a constellation of technical challenges in 2025, centered on data quality, model bias, and the escalating computational demands of high-resolution forecasting. The proliferation of dense sensor networks—from urban weather stations to remote IoT-enabled devices—has dramatically increased the quantity of available data. However, heterogeneity in sensor calibration, maintenance, and data transmission standards leads to persistent quality issues. Even major providers of meteorological instrumentation, such as Vaisala and Campbell Scientific, continue to refine guidance on equipment calibration and data validation, but inconsistencies across global networks remain a substantial barrier to robust micro-model performance.
Model bias in micro-scale NWP systems is another persistent challenge. Micro-models, by design, resolve atmospheric processes at scales of hundreds of meters or less, making them highly sensitive to land use, surface roughness, and local atmospheric chemistry. In urban environments, small errors in input data—such as underestimating heat fluxes from buildings or mischaracterizing surface moisture—can propagate into significant biases. Ongoing calibration efforts by agencies such as the European Centre for Medium-Range Weather Forecasts and the UK Met Office increasingly rely on data assimilation techniques and machine learning algorithms to mitigate systematic errors. Yet, the “ground truth” against which these models are calibrated is itself limited by observational gaps and sensor uncertainties.
The computational burden of micro-model calibration is intensifying as demand grows for real-time, hyperlocal forecasts. Simulating weather at fine spatial and temporal resolutions requires substantial processing power, especially when running ensemble forecasts or integrating large volumes of observational data. The transition to exascale computing, led by organizations like Cray (an HPE company) and IBM, is enabling more complex model physics and data assimilation schemes. However, even with these advances, balancing accuracy, run-time, and cost remains a core challenge. Cloud-based solutions offered by major cloud service providers are beginning to supplement traditional supercomputers, but questions about data security, latency, and reproducibility persist.
Looking ahead, the calibration of NWP micro-models will likely hinge on improvements in automated data quality control, advances in machine learning for bias correction, and continued investment in scalable computational infrastructure. Collaborative efforts between instrument manufacturers, national meteorological services, and computing hardware providers will be critical to overcoming these challenges and delivering the next generation of precise, reliable, and actionable weather forecasts at the micro-scale.
Competitive Landscape & Strategic Opportunities
The competitive landscape for numerical weather prediction (NWP) micro-model calibration in 2025 is characterized by rapid advancements in computational power, data assimilation techniques, and collaborative partnerships between meteorological agencies and technology firms. Key players such as European Centre for Medium-Range Weather Forecasts (ECMWF), UK Met Office, National Oceanic and Atmospheric Administration (NOAA), and IBM are at the forefront, leveraging high-performance computing and expanding observation networks to refine micro-model calibration.
In 2025, ECMWF is deploying its latest Integrated Forecasting System (IFS) upgrades, incorporating finer-scale local models and enhanced ensemble calibration, which significantly improve the accuracy of short-term and severe weather forecasts. Similarly, the UK Met Office has announced new deployments of its high-resolution convective-scale models, now calibrated with increased volumes of in-situ sensor and radar data, offering hyperlocal forecast improvements for urban and coastal regions.
Strategic partnerships are a hallmark of the current period. NOAA collaborates with private sector technology providers to integrate machine learning algorithms into model calibration pipelines, using real-time data feeds from both traditional meteorological instruments and non-traditional sources such as IoT weather sensors. Additionally, IBM continues to expand its The Weather Company offerings, focusing on AI-driven micro-model calibration and customized solutions for sectors like agriculture and renewable energy.
The emergence of commercial satellite constellations from companies like Spire Global and Planet Labs is further intensifying competition. These firms provide high-frequency, high-resolution atmospheric data that significantly enhance micro-model calibration, enabling forecasters to identify and adjust for local biases and microclimatic effects.
Looking ahead, strategic opportunities will center on the integration of heterogeneous data sources—including drone-based atmospheric profiling and crowd-sourced weather observations—into calibration workflows. Advances in explainable AI and quantum computing, under exploration by industry leaders, promise to unlock new horizons in model calibration precision and speed by 2027. Meteorological agencies and technology companies are expected to pursue joint ventures and open-data initiatives to standardize calibration protocols and share best practices, fostering a more competitive yet collaborative ecosystem.
Future Outlook: Disruptive Trends and Market Forecasts Through 2030
The landscape of numerical weather prediction (NWP) micro-model calibration is on the cusp of significant transformation as we move through 2025 and anticipate developments toward 2030. Micro-models—localized, high-resolution weather prediction models—have become increasingly essential for sectors ranging from precision agriculture to renewable energy and urban planning. The calibration of these models, which involves fine-tuning their internal parameters to better match observational data, is gaining momentum due to rapid advancements in data assimilation, machine learning, and sensor networks.
In 2025, a key trend is the integration of real-time, hyper-local data from dense sensor arrays, including next-generation weather stations, IoT-enabled devices, and remote sensing platforms. Companies such as Vaisala and Campbell Scientific are expanding their offerings of automated weather observation systems, which feed robust datasets into micro-models for calibration. This influx of granular data allows for more dynamic and adaptive calibration routines, improving model accuracy at the neighborhood or even street scale.
Machine learning is also emerging as a disruptive force. Major meteorological organizations like the European Centre for Medium-Range Weather Forecasts (ECMWF) are actively researching hybrid approaches that combine physics-based modeling with data-driven techniques for calibration and bias correction. Early deployments of these methods are demonstrating measurable gains in forecast skill and computational efficiency, especially in rapidly changing urban microclimates and complex terrains.
Looking ahead to the next five years, several disruptive trends are expected to shape the market. The proliferation of commercial nanosatellite constellations, led by companies such as Spire Global, is anticipated to yield high-frequency, high-resolution observational data. This will enable more frequent and precise recalibration of localized models. Furthermore, open data initiatives by agencies such as NOAA are expanding the availability of quality-controlled, near-real-time datasets, further empowering both public and private sector modelers.
The convergence of these trends is forecast to accelerate the adoption of micro-model calibration solutions across industries. By 2030, expect a marketplace characterized by automated, AI-enhanced calibration workflows, broader participation from non-traditional weather data providers, and a surge in demand for customized NWP services at micro-geographical scales. This evolution is likely to foster collaboration between hardware vendors, software developers, and end-users, fundamentally reshaping how localized weather insights are produced and utilized.
Sources & References
- European Centre for Medium-Range Weather Forecasts
- NVIDIA Corporation
- IBM Corporation
- IBM
- Developmental Testbed Center
- European Centre for Medium-Range Weather Forecasts
- Vaisala
- Meteomatics
- Netatmo
- WeatherFlow
- Deutscher Wetterdienst (DWD)
- Met Office
- Microsoft
- World Meteorological Organization
- Japan Meteorological Agency
- Campbell Scientific
- Cray (an HPE company)
- Planet Labs