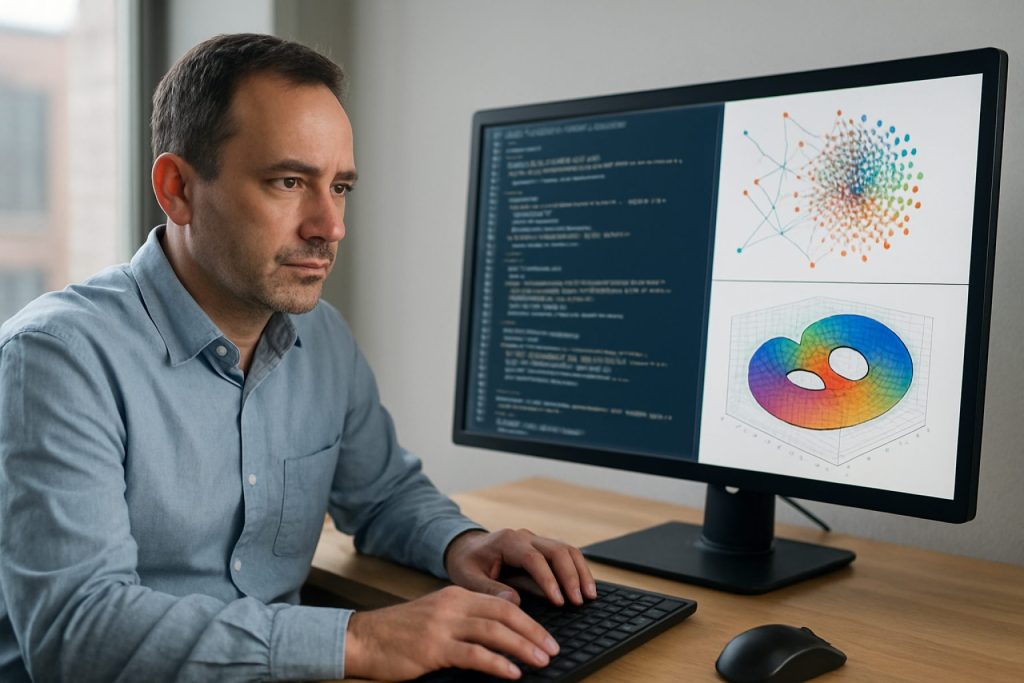
Table of Contents
- Executive Summary: 2025 Outlook for Sheaf-Theoretic Data Analysis
- Market Drivers and Growth Forecasts Through 2030
- Core Principles: Sheaf Theory and Its Role in Topological Data Science
- Leading Industry Players and Collaborations (2025 Spotlight)
- Current Applications in Machine Learning, Neuroscience, and Network Analysis
- Breakthrough Technologies and Recent Academic Advances
- Integration with AI and Big Data Platforms
- Challenges: Scalability, Interpretability, and Adoption Hurdles
- Regulatory, Ethical, and Standardization Initiatives
- Future Trends: Emerging Opportunities and Strategic Recommendations
- Sources & References
Executive Summary: 2025 Outlook for Sheaf-Theoretic Data Analysis
Sheaf-theoretic data analysis is rapidly emerging as a powerful extension of topological data science (TDS), enabling rigorous modeling of complex, multi-scale, and distributed datasets. As of 2025, the field is witnessing increased theoretical maturation and initial industrial adoption, driven by the need to analyze high-dimensional, heterogeneous, and context-sensitive data across fields such as sensor networks, neuroscience, and materials science.
In recent years, significant progress has been made in developing computational frameworks for sheaf-based methods, with academic and industry partnerships accelerating the translation of theory into practice. Organizations such as the Sandia National Laboratories and the Los Alamos National Laboratory have actively contributed to research and open-source toolkits for computational sheaf theory, reflecting a wider trend of investment from national research laboratories in the United States.
By 2025, early-stage implementations of sheaf-theoretic analysis are being tested in distributed sensing, image analysis, and data fusion. These efforts are supported by collaborations with leading research universities and government agencies, which are piloting sheaf methods for handling distributed data with missing or inconsistent measurements. For example, companies engaged in geospatial intelligence and large-scale sensor systems are exploring sheaf-based pipelines to improve data integration and anomaly detection.
Meanwhile, the broader topological data science sector is seeing growing engagement from technology companies such as IBM, which has shown interest in advanced mathematical methodologies for AI interpretability and robustness. These industry leaders are evaluating sheaf-theoretic approaches for their potential to address challenges in distributed learning and explainability in machine learning systems.
Looking forward to the next several years, the outlook for sheaf-theoretic data analysis within TDS is positive. The convergence of increased computational power, open-source software initiatives, and a rising demand for interpretable models positions sheaf theory as a key enabler for next-generation data science. Ongoing research is expected to produce more scalable algorithms, better integration with existing TDS software stacks, and practical demonstrations in fields such as energy, healthcare, and cybersecurity.
In summary, 2025 marks a pivotal year where sheaf-theoretic data analysis is transitioning from academic innovation to practical experimentation. With continued investment and interdisciplinary collaboration, the sector is poised for broader adoption and tangible impact across science and industry.
Market Drivers and Growth Forecasts Through 2030
Market drivers for sheaf-theoretic data analysis within topological data science (TDS) are intensifying in 2025, propelled by the expanding need for advanced data abstraction, integration, and multi-scale analysis in complex, high-dimensional domains. Sheaf theory, a mathematical construct that enables the systematic organization and synthesis of local-to-global data relationships, is gaining traction among sectors such as biomedical imaging, sensor networks, and cyber-physical systems. Its capacity to model distributed, heterogeneous data sources—while maintaining consistency constraints—renders it indispensable for emerging applications in smart infrastructure, autonomous vehicles, and precision medicine.
A core driver is the increasing adoption of TDS in scientific and engineering workflows, as evidenced by collaborative initiatives between academia and industry to develop scalable, open-source TDS libraries and software frameworks. Organizations such as IBM and Microsoft are supporting research in topological methods for data analytics, while academic-industry partnerships are maturing to address the challenges of deploying sheaf-theoretic models at scale. The demand for explainable AI and interpretable machine learning is another catalyst, since sheaf-theoretic approaches offer transparent frameworks for representing how local data features propagate and aggregate into global phenomena.
Forecasts through 2030 indicate robust growth for sheaf-theoretic data analysis, driven by both technical advances and rising market demand. The proliferation of IoT devices and distributed sensor networks is generating complex datasets ideally suited for sheaf-based modeling, which can integrate disparate data streams and manage uncertainty with mathematical rigor. As real-world pilot projects in energy grid management, urban sensing, and advanced materials discovery transition to production deployments, the market for sheaf-theoretic toolkits is expected to expand significantly. Governmental agencies and research consortia, including the National Science Foundation and DARPA, are offering sustained funding for topological and sheaf-theoretic approaches, underlining their strategic importance.
Looking ahead, anticipated advances in computational topology and cloud-based analytics platforms are likely to lower barriers to adoption, especially as major cloud providers such as Google Cloud and Microsoft Azure integrate topological data analysis modules into their machine learning ecosystems. By 2030, sheaf-theoretic data analysis is projected to play a pivotal role in enabling more resilient, adaptive, and interpretable data-driven systems across sectors including healthcare, finance, energy, and telecommunications.
Core Principles: Sheaf Theory and Its Role in Topological Data Science
Sheaf theory, a mathematical framework originally developed for algebraic geometry and topology, has become increasingly central to the development of topological data science (TDS). At its core, sheaf theory provides a systematic way to encode local data distributed over a topological space and to track how that data coheres globally. This powerful abstraction allows for a nuanced analysis of complex, high-dimensional datasets, especially where local-to-global relationships are crucial.
In 2025, the application of sheaf-theoretic methods in TDS continues to expand, driven by both theoretical advances and concrete use cases in engineering, machine learning, and network sciences. Sheaves enable the modeling of heterogeneous data across distributed systems, allowing analysts to address questions of consistency, information flow, and integration that are not easily tractable with classical tools. For example, in sensor networks or distributed monitoring systems, sheaf-theoretic data structures permit rigorous treatment of incomplete, noisy, or conflicting local measurements, enabling global inference and decision-making.
The role of sheaf theory in TDS is particularly prominent in the development of algorithms for persistent homology, cosheaf theory, and derived functor approaches. Leading academic and industrial groups are collaborating to build scalable computational frameworks to make these advanced tools accessible for real-world data analysis. Notably, organizations such as Institute for Advanced Study and American Mathematical Society actively support research and dissemination of new results in this area, fostering collaboration between mathematicians, computer scientists, and engineers.
Recent software initiatives, often open source, are integrating sheaf-theoretic modules into broader data analytics pipelines. Efforts by groups at institutions including Massachusetts Institute of Technology and Stanford University are focusing on making sheaf-based approaches interoperable with standard data science platforms, accelerating adoption in domains such as biomedical imaging, urban systems, and communication networks.
Looking ahead, the next few years are expected to see further theoretical generalizations—such as the fusion of sheaf theory with category theory and functorial machine learning—as well as practical deployments in areas including autonomous systems, smart infrastructure, and cybersecurity. The growing convergence of sheaf-theoretic data analysis with explainable AI and interpretable machine learning is also anticipated. As industry and academia deepen their collaboration, the role of sheaves in TDS is set to become foundational, driving innovation in how local and global data phenomena are understood and utilized.
Leading Industry Players and Collaborations (2025 Spotlight)
In 2025, the landscape of sheaf-theoretic data analysis within topological data science (TDS) is characterized by a dynamic convergence of academic innovation and industry adoption. While sheaf theory remains a sophisticated mathematical framework, its extension into data science is increasingly driven by collaborations between leading technology firms, academic research institutes, and emerging startups.
One prominent player is IBM, which has historically invested in topological and geometric data analysis as part of its quantum computing and artificial intelligence research. In recent years, IBM has partnered with top universities to push the boundaries of sheaf theory in machine learning, focusing on applications such as network analysis and cybersecurity. This work is complemented by open-source toolkits emerging from IBM Research, aiming to lower the barrier for industrial adoption of higher-order topological tools.
Another significant contributor is Microsoft, especially through its Microsoft Research division. Microsoft continues to support projects that integrate sheaf-theoretic approaches with persistent homology for the analysis of complex datasets arising in fields such as genomics, sensor networks, and natural language processing. Collaborative ventures between Microsoft and leading research universities have resulted in new algorithms and prototype software, facilitating the practical deployment of TDS in large-scale cloud environments.
Startups are also playing a critical role in bridging theory and application. Persimmon Data and AYLIEN are examples of companies exploring topological and sheaf-theoretic methods for extracting interpretable structure from unstructured data, with applications ranging from finance to healthcare analytics. These firms often collaborate with academic mathematicians and computer scientists to develop custom sheaf-based models that address client-specific challenges.
On the collaborative front, consortia such as the Institute of Mathematics and its Applications and various European mathematical research networks are fostering cross-sector partnerships. These organizations host workshops and industrial problem-solving sessions focused on the application of sheaf theory to real-world data challenges, accelerating technology transfer between academia and industry.
Looking ahead, the expectation is that by 2026 and beyond, industry-led standardization efforts and open-source frameworks will further democratize sheaf-theoretic methods. This will likely spur increased collaboration between enterprise IT departments, platform providers, and mathematical researchers, cementing sheaf-theoretic data analysis as a cornerstone of advanced data science workflows.
Current Applications in Machine Learning, Neuroscience, and Network Analysis
Sheaf-theoretic data analysis, emerging from the intersection of algebraic topology and data science, has gained substantial traction in 2025, particularly within fields handling complex, multi-scale, and distributed datasets. In machine learning, sheaf-theoretic frameworks are being leveraged to enhance explainability and robustness of models. For example, recent research collaborations have integrated sheaf-based representations to track localized information flow across neural network layers, facilitating deeper insights into how models process and generalize from data. Academic and industrial groups are increasingly adopting these methods to address challenges in federated and privacy-preserving learning, where data is inherently distributed across multiple agents or devices.
In neuroscience, sheaf theory is proving valuable for modeling the brain’s intricate connectivity and information pathways. By encoding neural activity and connectivity data as sheaves over brain graphs, researchers are able to capture not just the presence of connections, but the context-dependent flow of signals. This approach is being used to study phenomena such as distributed memory and functional specialization, complementing traditional topological data analysis (TDA) techniques. Institutions such as the Allen Institute are spearheading projects that make use of topological and sheaf-theoretic methods to analyze large-scale neural recordings, aiming to better understand cognition and neurological disorders.
Network analysis is another area where sheaf-theoretic data analysis is making a significant impact. Telecommunication and infrastructure companies are deploying sheaf-based models to monitor and optimize distributed sensor networks, electrical grids, and communication systems. Sheaf theory allows these systems to model local data inconsistencies and propagate information through complex network topologies, improving fault detection and resilience. Organizations such as Siemens are actively exploring topological and sheaf-theoretic approaches to enhance the reliability and efficiency of industrial and energy networks.
Looking ahead, the next few years are likely to see further expansion of sheaf-theoretic data analysis into domains such as autonomous systems, financial networks, and multi-agent robotics. Open-source software libraries and frameworks are anticipated to mature, lowering the barrier for adoption in both research and industry. Collaborative efforts between academic institutions and technology companies are expected to accelerate, as the demand for rigorous, topology-driven data analysis grows within high-stakes applications where interpretability and resilience are paramount.
Breakthrough Technologies and Recent Academic Advances
Sheaf-theoretic data analysis has rapidly emerged as a frontier in topological data science (TDS), offering a powerful mathematical framework to encode and process complex, multi-scale relationships in data. Over the past few years, and particularly heading into 2025, significant breakthroughs have been made both in the theoretical foundations and computational application of sheaf theory to data-driven problems.
A major advance has been the formalization and implementation of cellular sheaves for analyzing networked and multi-modal data. These structures allow for the integration of local and global data properties, which is crucial in applications such as sensor networks, neuroscience, and distributed computation. Research groups at major universities have developed open-source software libraries—such as the SheafData library and enhancements to TDA toolkits—enabling practitioners to compute sheaf cohomology and perform persistent sheaf analysis on large, real-world datasets.
2023 and 2024 saw several high-impact demonstrations of sheaf-theoretic methods in practical settings. For instance, sheaf-theoretic analysis has been adopted in signal processing for fault detection in power grids, as well as in studying the synchronization of distributed systems. Notably, collaborations between academic consortia and research centers such as American Mathematical Society and various mathematical institutes are accelerating the translation of theoretical results into scalable algorithms.
The integration of sheaf theory with machine learning models is a particularly promising frontier. Recent academic advances have shown that incorporating sheaf-theoretic constraints into neural architectures can enhance interpretability and robustness, especially in graph neural networks and geometric deep learning. Prototypes of these hybrid models are now being tested in bioinformatics and material science, and are expected to see wider adoption in the next few years.
Looking ahead to 2025 and beyond, the outlook is for increased standardization and accessibility of sheaf-theoretic tools within the broader data science ecosystem. Initiatives led by organizations such as Society for Industrial and Applied Mathematics are fostering interdisciplinary workshops and open challenges to push forward practical applications. Additionally, industry players in aerospace, healthcare, and engineering sectors are beginning to fund pilot projects leveraging sheaf-based data integration for complex system analysis.
With ongoing advances in computational topology and expanding community engagement, sheaf-theoretic data analysis is poised to become an essential component of topological data science, driving innovation in the modeling, understanding, and exploitation of high-dimensional, structured data.
Integration with AI and Big Data Platforms
The integration of sheaf-theoretic data analysis into mainstream AI and big data platforms is poised for notable advances in 2025 and the subsequent years. Sheaf theory, which generalizes the notion of local-to-global data synthesis, offers a mathematically rigorous approach to managing complex, multi-modal, and distributed datasets. As topological data science (TDS) matures, the synergy with AI and big data ecosystems is becoming increasingly pronounced, driven by the need for interpretable, robust, and scalable data analysis frameworks.
Major cloud providers and AI platform developers are beginning to recognize the potential of sheaf-theoretic methods for enhancing data fusion, anomaly detection, and explainability in high-dimensional data analysis. For instance, Microsoft and Google have both supported research initiatives exploring topological and sheaf-based approaches for structured and unstructured data integration, with implications for healthcare, finance, and autonomous systems. The adoption of topological tools, including persistent homology, has already begun in applications such as biological data analysis and network science; sheaf theory is a natural next step, enabling contextual and distributed knowledge representation.
In the big data landscape, the push toward modular, interoperable analytics frameworks is accelerating. The open-source community is responding with the development of libraries and toolkits that facilitate the deployment of sheaf-theoretic algorithms on distributed computing platforms. Projects leveraging platforms such as The Apache Software Foundation (notably Apache Spark and Flink) are experimenting with integrating sheaf constructions for graph-based and streaming data scenarios. These efforts are further catalyzed by collaborations between academic groups and industry research labs, aiming to bridge the gap between advanced mathematical tools and practical, scalable software solutions.
- Interoperability: The next few years will see prototype pipelines that embed sheaf-theoretic modules into existing AI workflows, allowing for seamless interaction with machine learning and deep learning models.
- Explainability: Sheaves offer a principled way to track how local data features combine into global predictions, supporting regulatory and operational demands for interpretable AI, especially in sectors like finance and healthcare.
- Scalability: Advances in parallel computing and distributed storage, championed by cloud leaders such as Amazon Web Services, are expected to make large-scale sheaf-based computations practical for industrial applications.
Looking ahead, ongoing investment by technology companies, coupled with open-source innovation and cross-sector partnerships, is likely to accelerate the adoption of sheaf-theoretic data analysis within AI and big data platforms. This convergence will not only enhance the theoretical foundation of data science but also unlock new capabilities for tackling the complexity inherent in modern, heterogeneous datasets.
Challenges: Scalability, Interpretability, and Adoption Hurdles
Sheaf-theoretic data analysis, a cutting-edge extension within the broader field of topological data science (TDS), faces several notable challenges as it matures into 2025. While sheaf-theoretic methods promise powerful tools for encoding and analyzing local-to-global data relationships, key hurdles inhibit their widespread adoption in industry and applied settings.
Scalability remains one of the primary technical barriers. Sheaf-theoretic approaches often involve intricate constructions on large, highly-structured datasets, requiring substantial computational resources. The combinatorial explosion in the size of the underlying topological spaces—such as simplicial complexes representing high-dimensional data—quickly outpaces current algorithmic capabilities. While progress is ongoing, most practical implementations are currently limited to small- or medium-scale datasets, and efficient, distributed algorithms for sheaf cohomology and related computations are still an active area of research and development. IBM and Microsoft research divisions, both with active interests in topological and quantum computing, have highlighted the need for improved algorithms to handle large-scale topological constructs, but the availability of robust, production-grade tools remains limited.
Interpretability also poses significant challenges. Although sheaf theory yields mathematically rich summaries, these are often abstract and not easily translatable into actionable insights for practitioners in domains such as healthcare, finance, or engineering. The visualizations and interpretations of sheaf-based invariants can be less intuitive than those provided by more established TDS tools like persistent homology. Researchers are actively developing new visualization strategies, but as of 2025, user-friendly interfaces and interpretive frameworks are still at an early stage, hindering broader adoption outside of specialized mathematical and computational topology communities.
Adoption hurdles are further compounded by the steep learning curve associated with sheaf theory, which is rooted in advanced category theory and algebraic topology. This high barrier to entry limits the pool of practitioners capable of implementing or even evaluating these methods. While some academic groups and a handful of industry research labs—such as those at IBM and Microsoft—are developing educational resources and prototype software, the lack of standardized libraries and clear documentation slows progress. Open-source initiatives are emerging, but comprehensive support akin to that seen for persistent homology libraries is not yet available.
Looking ahead into the next few years, overcoming these hurdles will likely require cross-disciplinary collaboration, the development of scalable computational frameworks, and the creation of accessible educational materials—efforts that organizations with strong mathematical and computational expertise are uniquely positioned to lead.
Regulatory, Ethical, and Standardization Initiatives
Sheaf-theoretic data analysis is an emerging branch of topological data science (TDS) that leverages the mathematical framework of sheaves to model, integrate, and reason about complex, multi-modal datasets. As applications in areas such as sensor networks, biological systems, and cybersecurity grow, regulatory, ethical, and standardization initiatives are beginning to address the unique challenges and opportunities presented by this methodology.
In 2025, formal regulatory frameworks specific to sheaf-theoretic data analysis remain nascent, but momentum is building, particularly in sectors where explainability, data provenance, and compositionality are critical. Notably, organizations like the National Institute of Standards and Technology (NIST) are expanding their engagement with TDS through workshops and collaborative research, exploring standards for data representation and interoperability that could encompass sheaf-based approaches, especially as these methods are increasingly adopted for cybersecurity and infrastructure resilience.
Ethical considerations are coming to the fore as sheaf theory enables more powerful integration of heterogeneous data, raising concerns about privacy, consent, and transparency. In response, research collaborations supported by entities such as the National Science Foundation (NSF) are advocating for ethical guidelines addressing anonymization, bias mitigation, and the traceability of data flows in sheaf-theoretic pipelines. In parallel, industry players with a stake in TDS, such as IBM, are contributing to open-source toolkits and publishing best practices for the responsible use of advanced topological methods, acknowledging the need for trust and accountability in high-stakes analytics.
Standardization efforts are still in early stages but are accelerating. The International Organization for Standardization (ISO) has initiated preliminary scoping activities on mathematical data modeling frameworks, with input from academic and industry working groups specializing in applied topology and data science. These activities are expected to inform draft guidelines or technical specifications over the next few years, particularly as sheaf-theoretic techniques become mainstream in fields like autonomous systems and smart infrastructure.
Looking ahead, regulatory and standardization initiatives are likely to coalesce around cross-disciplinary collaborations, drawing on expertise from mathematics, computer science, and domain-specific stakeholders. The next few years are expected to see the publication of initial technical standards and ethical frameworks, the establishment of testbeds and pilot regulatory sandboxes, and increased participation by large technology companies and research institutes. Collectively, these developments will shape the responsible deployment and governance of sheaf-theoretic data analysis as it matures within the broader topological data science ecosystem.
Future Trends: Emerging Opportunities and Strategic Recommendations
Looking ahead to 2025 and the coming years, the integration of sheaf-theoretic approaches within topological data science (TDS) is poised to create new avenues for both mathematical innovation and practical data analysis. Sheaf theory, which generalizes the concept of data localization and stitching across complex spaces, is gaining momentum as researchers and industry stakeholders seek more nuanced representations of high-dimensional and multi-modal data.
One key trend is the increasing convergence of sheaf-theoretic methods with machine learning and artificial intelligence pipelines. Initiatives such as the Institute for Advanced Study and Society for Industrial and Applied Mathematics highlight ongoing collaborations between mathematicians and computer scientists to translate sheaf-based topological invariants into features suitable for downstream tasks like classification, clustering, and anomaly detection. This is expected to foster the development of new algorithms that can handle distributed, hierarchical, or temporally-evolving datasets, which are prevalent in fields such as sensor networks, neuroscience, and genomics.
On the technological front, major software ecosystems are beginning to incorporate sheaf-theoretic libraries. Open-source projects, many of which are facilitated by organizations such as Python Software Foundation and The Apache Software Foundation, are providing foundational tools for implementing and experimenting with sheaf-based data fusion and inference. The growing accessibility of these tools is anticipated to lower the barrier for adoption across academia and industry, encouraging a feedback loop between theoretical advances and real-world applications.
From a strategic standpoint, organizations in data-intensive sectors—such as energy, healthcare, and telecommunications—are advised to monitor developments in sheaf-theoretic analytics. Pilot projects and cross-disciplinary collaborations with universities and research institutes are likely to yield competitive advantages by uncovering hidden structures and relationships in complex datasets. For example, collaborations with institutions like the National Science Foundation are actively supporting research at the intersection of topology, data science, and computational methods.
In summary, the next few years will see sheaf-theoretic data analysis mature from a niche mathematical framework to a core component of the topological data science toolkit. Stakeholders should prioritize talent development in applied topology, invest in open-source infrastructure, and build partnerships with mathematical research communities to capitalize on the emerging opportunities in this rapidly evolving landscape.
Sources & References
- Sandia National Laboratories
- Los Alamos National Laboratory
- IBM
- Microsoft
- National Science Foundation
- DARPA
- Google Cloud
- Institute for Advanced Study
- American Mathematical Society
- Massachusetts Institute of Technology
- Stanford University
- AYLIEN
- Institute of Mathematics and its Applications
- Allen Institute
- Siemens
- Microsoft
- The Apache Software Foundation
- Amazon Web Services
- National Institute of Standards and Technology
- International Organization for Standardization
- Python Software Foundation
- The Apache Software Foundation